How Does a Dataset Become a Data Product?
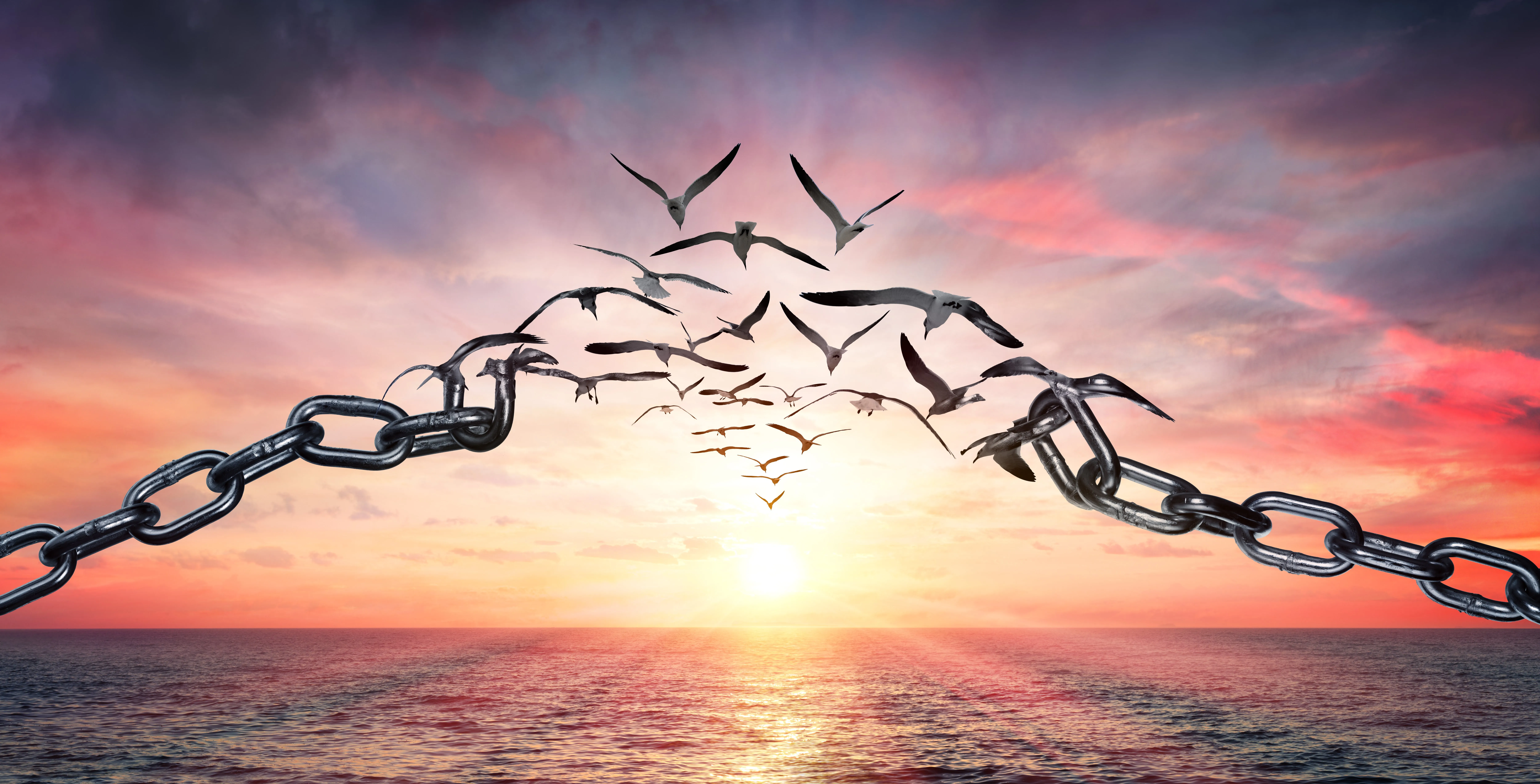
We know that a data product is the output created after processing, analysis, and interpretation of raw data. But what, exactly, does that entail? What happens to a raw dataset on that journey to becoming a refined and actionable data product? In the second of our series on data products, we’ll explore the steps involved in this transformation, shedding light on how data—sometimes considered a passive resource—can become a dynamic driver of decisions and innovation.
Step 1: Start with the raw dataset
The process of building a data product begins with selecting the raw dataset—a collection of structured or unstructured information that is a repository of potential knowledge. This raw data could be sourced from various channels, including internal databases, external APIs, sensor data, or myriad sources depending on the data producer’s industry or the objectives of the data product. With a specific dataset selected, we then take a closer look to understand its granularity, quality, and other characteristics; this will give us the foundation we need to make the right decisions in the future when processing the dataset.
Step 2: Data collection and cleansing
Next, data collection and cleansing is performed to transform the raw data into a clean and reliable dataset, ready for further analysis and transformation. The data cleansing process entails identifying relevant data sources, ensuring data accuracy, dealing with missing values, and handling inconsistencies. This groundwork is essential to prevent biases and inaccuracies down the line that could compromise the integrity of the final data product.
Step 3: Processing and analysis
With a refined dataset in hand, the next step involves using advanced processing and analysis techniques. Machine learning algorithms, statistical models, and other analytical methods come into play to extract meaningful patterns, trends, and insights from the data. This phase is crucial for unlocking the latent value within the dataset, turning it into actionable intelligence.
Step 4: Visualization to make information accessible
Our raw data has been collected, cleansed, harmonized, and otherwise processed for consumption. Now, we must ensure that the developing data product includes some visualization so data consumers can more easily understand what they’re looking at. Adding in the capabilities to produce infographics, charts, and interactive dashboards means that complex data can be transformed into an output that is more digestible. This not only facilitates understanding among non-technical stakeholders but also aids in effective decision-making, enabling users to understand—at a glance—sales trends, market performance, customer demographics, or any number of other metrics.
Step 5: Integration into the ecosystem
Our dataset is now nearly complete; at this point, it needs to be integrated into existing systems and workflows so that any of the resulting information can contribute to the broader organizational ecosystem. This phase involves linking the data product with relevant applications, databases, or other tools, creating a cohesive environment for data-driven decision-making.
Step 6: Ensuring results are democratized
To maximize the utility of a data product, it should be accessible to a wide range of stakeholders, not just data scientists or analysts. Consequently, creating a user-friendly interface is instrumental when it comes to data democratization. By adding in intuitive tools or capabilities, users with varying technical backgrounds will all be able to interact with and derive value from the data product with the same level of success.
For example, a be user-friendly sales dashboard derived from a customer relationship dataset can enable sales representatives to easily track performance, identify trends, and tailor their approaches to individual customers. In healthcare, a user-friendly interface empowers clinicians to make data-informed decisions regarding patient care without requiring extensive—and time-consuming—technical training.
Step 7: Continuously refining and improving
Our raw dataset is now a data product, and it will from here on out be subjected to an ongoing process of refinement and improvement so that it will remain relevant, accurate, and aligned with the organization's goals. These adjustments will vary depending on what kind of data is being used and what it’s being used for, but user feedback will ensure that the data product, rather than being a static output, is a dynamic and responsive tool that evolves over time.
From potential knowledge to tangible value
Each stage of the data product creation process is crucial for successfully transforming a raw dataset into a user-friendly and impactful product. Combining cleansing, harmonization, visualization, and a user-friendly interface, a well-designed data product both informs daily business decisions and drives organizations forward to greater innovation and more strategic business planning.