A 2023 Content Retrospective - Embracing Generative AI and LLMs
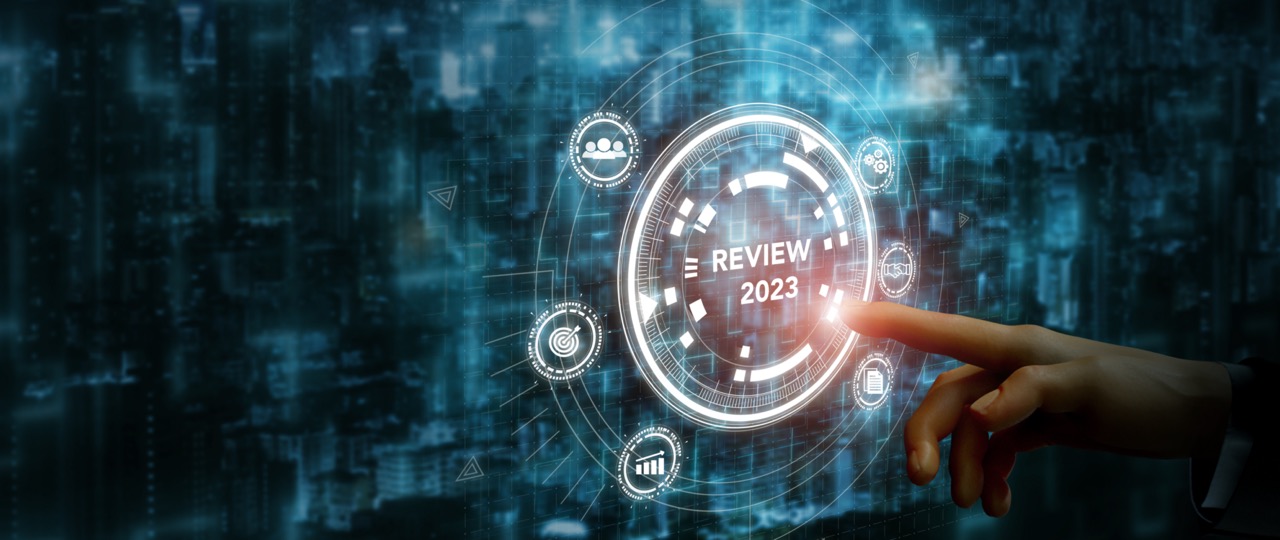
By Vasu Sattenapalli, CEO at RightData.
What a year it's been! At RightData, we’ve made some serious strides in the data landscape, and I'm thrilled with the progress. We've embraced generative AI and LLMs to shake up the data management scene. No more exclusive club for the techies —now everyone, regardless of tech skills, can dive into those complex data sets. It's all about asking questions in plain language and letting those LLMs do their magic.
We've not only torn down the barriers but also supercharged our decision-making process. Of course, change always brings challenges, and we've been on top of it—tackling issues from privacy concerns to biased results. Our focus has been on adding real value, not just chasing the shiny new tech.
If you’re interested in reading more of my opinions, I’ve rounded up all of the articles with my byline this year. I’m really proud of this work! Here's to a year of democratizing data and staying ahead in the game!
Are CDOs Too Focused on Data Silos? (Spiceworks)
Companies, regardless of size, grapple with scattered data silos, duplication, and trust issues. Traditional solutions like data warehouses overlook the value of data duplication and existing architectures. The recommended approach involves building a transparent framework for understanding duplicate data, context, and trust metrics.
3 Reasons Why Data Integrity is Everyone’s Biggest Data Challenge (RT Insights)
As modern data stacks become more complex, companies face challenges in maintaining data integrity throughout the data life cycle. Issues include the multitude of transformations data undergoes, migration to modern platforms without ensuring trust, and the struggle of data innovations to match fast-changing business needs.
3 Keys to Making Data Democratization a Reality (InfoWorld)
Executives struggle with the reality of data democratization as only 32% of available enterprise data is utilized, posing a threat to business agility. Addressing these challenges requires a strategy focusing on accessible data products, trust in data, and no-code options. Yet data democratization hinges on users finding, accessing, and trusting data, with an accessible data product offering parameters tapping into metadata.
Why Is Data Quality Still So Hard to Achieve? (Dataversity)
Despite the abundance of data tools and awareness, achieving data quality remains challenging due to exploding data volume, persistent silos, and the lack of a true data culture. The sheer volume and diversity of data, estimated to reach 180 zettabytes by 2025, create challenges in maintaining consistent data quality standards. To reconcile these issues, a three-pronged approach is recommended: improve the data itself with automation, facilitate cross-silo data use through catalogs and integrity checks, and build a data-focused culture with education and governance.
Are You Making Decisions Based on Bad Data? (Spiceworks)
Amidst the increasing reliance on data, achieving good data quality remains challenging. Recognizing symptoms of poor data, such as errors, integration issues, user feedback, and frequent data cleansing, is crucial. To address these issues, a three-pronged approach is recommended: Give assets appropriate weight during quality checks to prioritize critical domains; Adjust data governance processes regularly to align with evolving data landscapes; and use systems with built-in data quality processes, exploring data products and automated software for unified, structured, and accurate datasets. This proactive approach enables organizations to navigate challenges and enhance confidence in decision-making.
How Generative AI Will Empower Self-Service Data Management (BuiltIn)
Generative AI, powered by large language models (LLMs), is poised to democratize data management by enabling non-technical users to navigate complex data sets independently. LLMs, with billions of parameters, bridge the gap between intricate data structures and users lacking technical expertise. Users can pose natural language queries, allowing interactive exploration and insights. This transformation challenges traditional data management dominated by technical experts, offering a more inclusive approach. Implementing generative AI requires addressing change management, privacy concerns, biased results, and ongoing model training.