Data Validation to Ensure Internal Data Integrity at EY
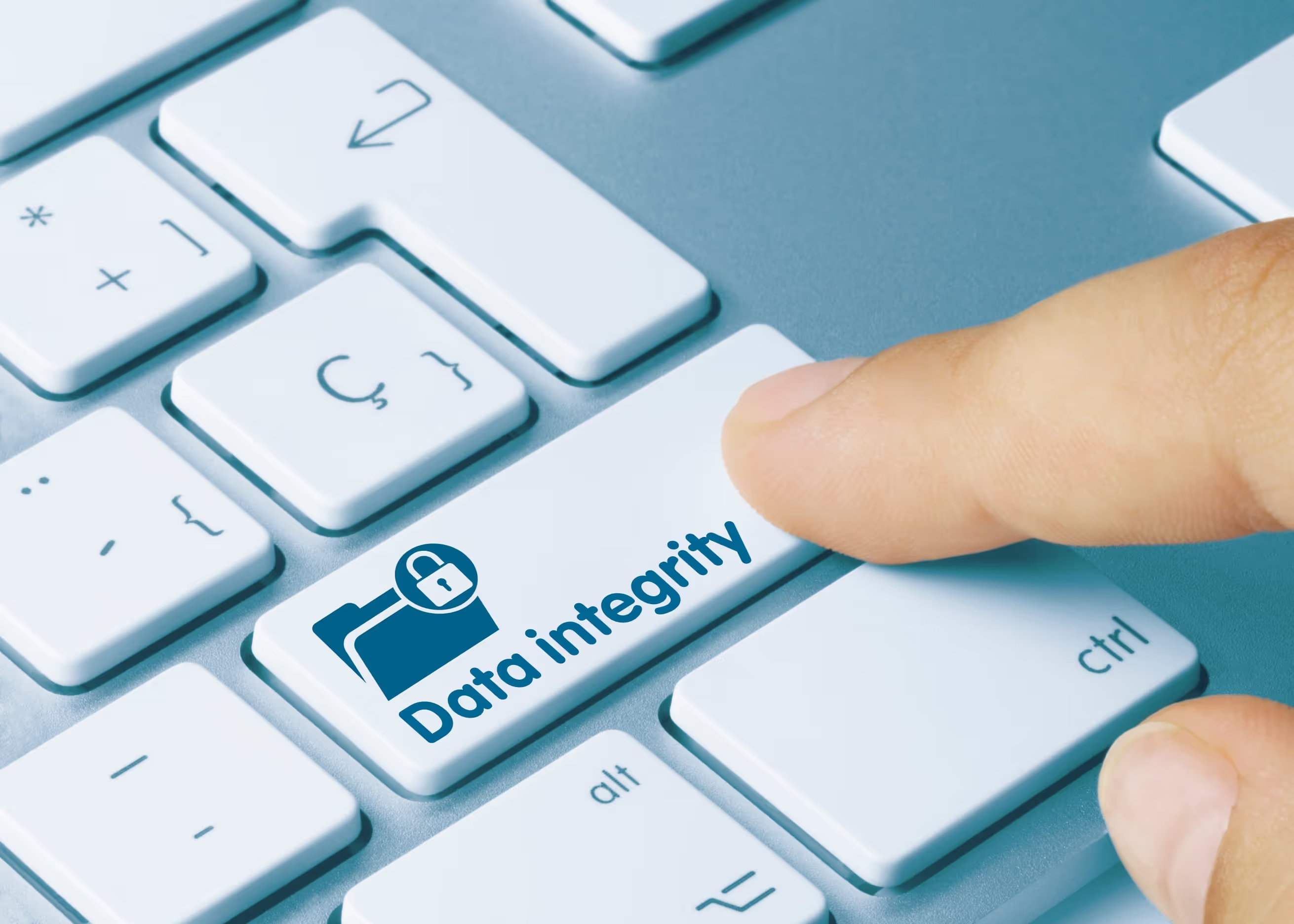
The Need
Ernst & Young, or EY, is one of big four professional services networks in the world providing financial audit, tax, consulting, and advisory services to its clients. Each large practice depends on data teams to collect and manage internal financial performance data that is validated and useful for final internal reporting. In addition, internal governance and compliance depend on a robust data quality process to verify there are no major errors in the data sets provided to EY’s many departments.
With current data workflows in place, a further layer of protection was needed to check both the logic and completeness of data being fed into the system, which is integral to devops. Multiple small projects with disparate data required validation to ensure the SQL database in Azure was normalized and correct for both structure and logic. Internal clients depend on this process to ensure both the financial reporting and the data supporting it are correct.
The Solution
RightData's DataTrust software (formerly called DataTrust) was deployed to automatically validate the large volume mix of disparate data, projects, and department reporting across the enterprise. Of special importance was reconciliation where FDR (Functional Data Reconciliation) built the scenarios within DataTrust to test the data based on application functionality of the application and was compared to a pair of datasets on a field-by-field level. Further, the TDR (Technical Data Reconciliation) helped to compare bulk datasets to do the sanity checks for the key attributes, like the number of the tables, record count from each table, metadata comparison, etc. This created an end-to-end cycle that assisted as data was fed into PowerBI reporting.

Impact
As one of the most trusted worldwide companies, that provide audit and tax assurance to major clients, EY also makes an internal commitment on providing high quality and trustable data for internal clients. The impact of validation is primarily saving precious time and money; but more it is the stalwart knowledge that internal data processes are in place to advise if there are any irregularities or deeply impacting logic mistakes. DataTrust provides that guarantee and gives both an operation and audit edge to the internal data team at EY.
The RightData Edge
Without question, data validation unifies data understanding for ingested data, warehouse data, and data fed into reporting. The edge that RightData provided was its know-how on each specific internal department use case or project – each had its own nature and with DataTrust, the EY internal data team could provide a consistent approach for its enterprise. An EY manager stated, “RightData understood the importance of our data validation operation and was there with both advice and software knowledge when it counted.”
Learn more about DataTrust
DataTrust is a comprehensive platform for data quality, risk, or compliance needs. Learn more or contact us to chat about your needs.
DataTrust Data Quality: A no-code data quality suite that improves data quality, reliability, consistency, and completeness of data. Data quality is a complex journey where metrics and reporting validate their work using powerful features such as:
Database Analyzer: Using Query Builder and Data Profiling, stakeholders analyze the data before using corresponding datasets in the validation and reconciliation scenarios.
Data Reconciliation: Comparing Row Counts. Compares number of rows between source and target dataset pairs and identifies tables for the row count not matching.
Data Validation: Rules based engine provides an easy interface to create validation scenarios to define validation rules against target data sets and capture exceptions.
Connectors For All Type of Data Sources: Over 150+ connectors for databases, applications, events, flat file data sources, cloud platforms, SAP sources, REST APIs, and social media platforms.
Data Quality: Ongoing discover that requires a quality-oriented culture to improve the data and commit to continuous process improvement.
Database Profiling: Digging deep into the data source to understand the content and the structure.
Data Reconciliation: An automated data reconciliation and the validation process that checks for completeness and accuracy of your data.
Data Health Reporting: Using dashboards against metrics and business rules, a process where the health and accuracy of your data is measured, usually with specific visualization.