Evolution of the Modern Data Stack: From ETL to ELT to ELTT
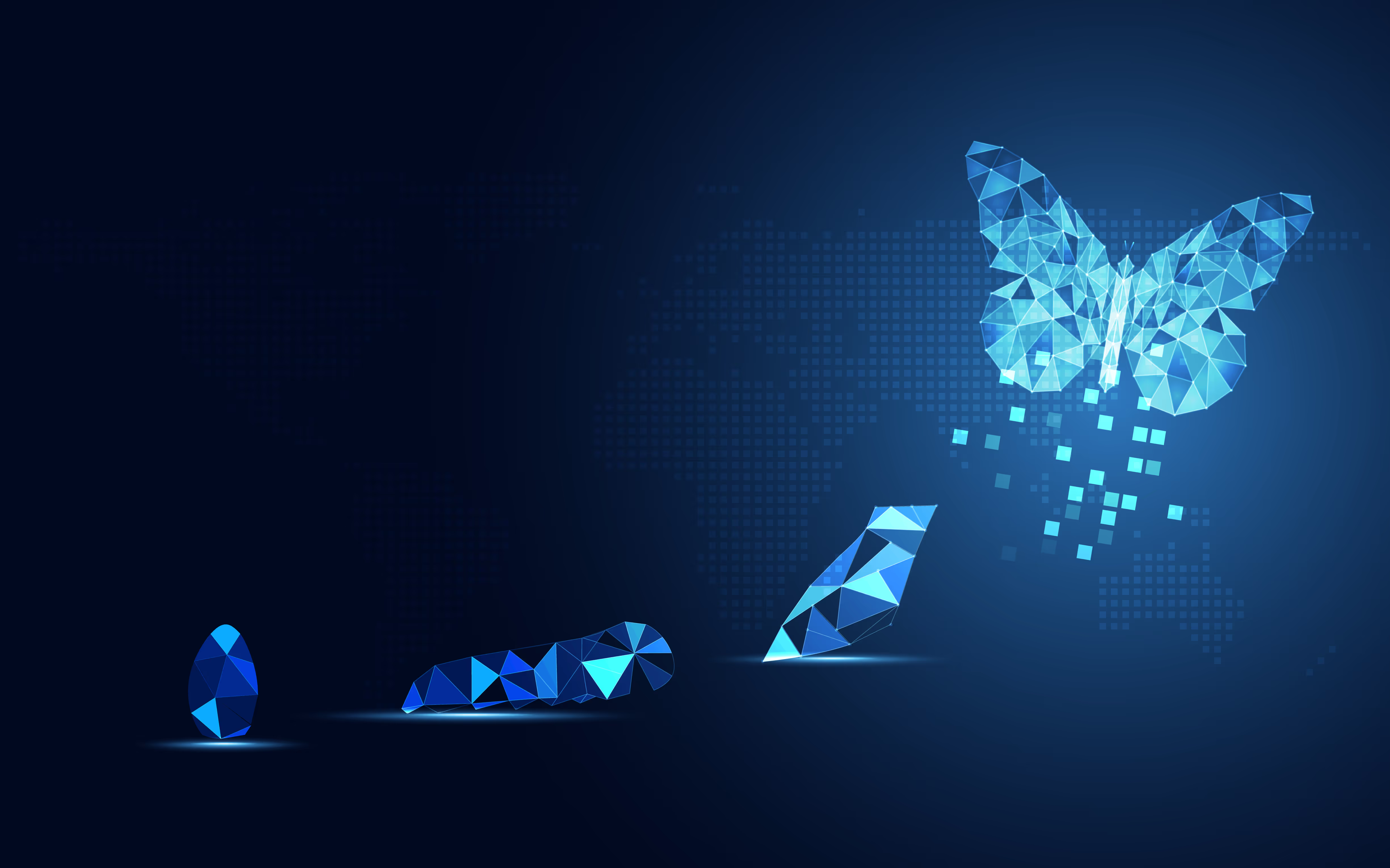
Due to the ever-evolving nature of data management, the modern data stack has undergone dramatic changes over the past few decades. One of those major updates involves the evolution of the data integration process. Historically, extract, transform, load (ETL) was the backbone of data warehousing until the advent of cloud computing led to a shift toward extract, load, transform (ELT). Now, we’re seeing the next phase data integration begin with the dawn of ELTT, which stands for extract, load, transform, trust. ELTT goes a step further, improving upon the previous advantages of ELT while providing users with an added layer of transparency and data reliability.
From Traditional ETL to Modern ELT: A Key Evolution in Data Integration
For decades, ETL was relied upon primarily because data warehouses couldn't yet handle large-scale transformations. In the ETL approach, data is first extracted from the source systems, then transformed or cleansed according to predefined business rules or data models, and finally loaded into the target data storage. While ETL was a helpful process for organizations looking to centralize data from different sources into a consistent format, the process was generally time-consuming, especially when large volumes of data needed to be transformed prior to loading.
Eventually, the sources of data and the target databases moved to the cloud. As cloud data warehouses became more common, the ELT approach gained popularity. By flipping the transform and load phases, organizations were able to directly transfer raw or minimally processed data into the data warehouse or data lake, and then leverage the more robust processing capabilities of cloud data warehouses to allow for more efficient and scalable data processing.
The advantages—and limitations—of ELT
On the whole, ELT represents a significant improvement over ETL. By loading the raw data first, organizations can quickly ingest and store massive volumes of data without the time and expense of conducting complex transformations upfront. It also allows for greater flexibility and agility in working with diverse data sources, and it eliminates the need for a separate server to handle data transformations. In addition, ELT enables exploration and analysis on raw data, providing opportunities for advanced analytics and machine learning on the entire dataset.
However, it's important to note that ELT still has its limitations. Because transformations are performed once the data has been ingested into the data warehouse, there is a risk of exposing raw, sensitive, or inaccurate data to end-users.
Adding trust to the equation with ELTT
So while ELT solves many of the inefficiencies of ETL, it still lacks an essential component: ensuring that the data we rely on is accurate and trustworthy. This is crucial for businesses that base their decisions on data, as even the slightest inaccuracy can have significant repercussions, ranging from poor business decisions to lack of adoption—and subsequent lack of ROI. By adding in a focus on trust with ELTT, organizations can enable the efficient processing of data as well as its integrity, security, and reliability.
- Data integrity: Because data is extracted and loaded directly without immediate transformations, it's important to have confidence in the correctness, completeness, and consistency of the data being ingested. Organizations need to establish mechanisms to validate and verify their data during and after the loading phase to maintain data integrity.
- Data security: Taking data from disparate sources and loading it into a central repository can introduce potential security risks. Implementing robust security measures, such as encryption, access controls, and monitoring mechanisms, helps build trust in the security of the data throughout the ELTT process.
- Compliance and privacy: Trust is closely tied to compliance with data protection regulations and privacy requirements. Organizations must ensure that the ELT process involves implementing appropriate data governance practices, providing transparency in data handling, obtaining necessary consents, and safeguarding personal or sensitive information.
- Data source reliability: Organizations need to evaluate and verify the credibility of the data sources they are extracting from, ensuring that the data is accurate, up-to-date, and fit for the intended purpose. Establishing trust in the reliability of data sources helps avoid propagating errors or inconsistencies during the ELTT process.
- Transformation validations: When performing transformations within the target storage, it is crucial to validate the transformations applied to the data. Trust can be built by implementing validation mechanisms, conducting data quality checks, and comparing the transformed data against predefined business rules or expectations.
By instilling trust throughout the ELT process, organizations can have confidence in the data they are leveraging for decision-making, analytics, and other downstream applications.
Streamline data processing and ensure data trustworthiness
As the data landscape continues to evolve, it is essential for businesses to adapt to these changes. The shift from ETL to ELT was just the beginning. With ELTT, businesses can now ensure that their data is not just processed efficiently but is also reliable and accurate.
RightData is at the forefront of this transformation. While other tools might require you to purchase an entirely different solution to enable data transparency and trust, RightData includes it as part of the package. By integrating data observability into the ELT process, RightData effectively offers a “modern data stack in a box,” ensuring that your data is monitored for quality and accuracy so you can make informed decisions in real time.