The 5 W’s of Metadata
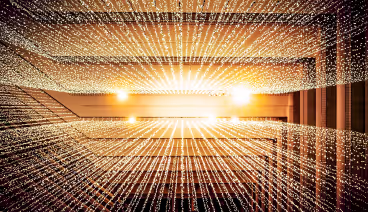
Thinking About the Value of Metadata
Metadata is “data about data.” It provides additional context about data, such as the name, data type, relationship to other data, definition of the data individual element, data mappings, and data transformation rules. Like an iceberg, there is quite a bit of understanding for what is seemingly only a data asset.

For true operational value, metadata must be part of a modern workflow, where solutions for cloud, APIs, and other federated data products need context and lineage from metadata. In addition, as part of a new wave of data catalog management, modern metadata must enable collaboration for all data users. Metadata itself continues its growth with datasets that are searchable and ready to be analyzed.
Fundamental Metadata
For the data scientist, taking a look from three perspectives provides a foundation to understand the value of metadata, shown below in three areas.
- Business – Includes the business glossary, business rules and algorithms, data lineage, data quality indicators (such as completeness and freshness indicators), ownership/stewardship information, logical data models, concept models, tagging, and certification.
- Technical – Includes database, tables, columns, indexes, referential integrity information, physical data models, and canonical information models.
- Operational – Includes data lineage, audit control information, run-books/playbooks, and policies.
What Metadata Gives You
Data practitioners – from data scientists to business users – must assess what they need from metadata as they are a critical part in managing the data product. In effect, they get telemetry from logical data workflow so they can optimize performance of the architecture and make changes as new assets arrive. This approach promotes the deep use of enterprise data catalogs to support evolving DataOps environments as well.
What To Think About Initially
- Diversity of metadata and data. Both operations and development leaders know that assets, policy, and code have to be unified with a concept of a data product, and a lifecycle that manages the process over time.
- Unified collaboration and transparency between DevOps and DataOps. All workflows should be bi-directional and metadata management is core to the test and build work loop.
- Value to machine learning. With a greater need for data wrangling that feeds an up-to-date model, metadata management can guide the steps to stay ahead of complex learning – unsupervised or supervised.
- Data quality. Because metadata deeply characterizes data, it determines the operational control need for quality governance, audit, and compliance. In fact, creating more trusted data becomes the goal foundation of the 5 W’s of metadata and its relevance to better data enterprise operations.

The 5 W’s of Metadata. Plus One More.
Great data scientists are always asking questions to start and since business needs can be met with the right architecture and technology implementation, the 5 W’s of metadata management are a good place to start. Shown below are the key questions.
Moving down each column for each W (with a bonus How), data scientists can ensure they have metadata management covered, as well as collaborating across teams.

Technology Augmented Curation
Does the naming of data assets and taxonomy matter? Decidedly yes. Decision-making can be dramatically impacted through better metadata management; and technology can augment the curation of metadata quickly and effectively to speed the process.
First, as organizations grow into more cloud data repositories, they simplify the ingest and storage to move beyond analyzing structured data assets (customer data, invoices, inventory, finance).
Today, the ability to extend and augment with unstructured data assets (e.g. emails, customer feedback, weblogs, call center info) is a major requirement for complete understanding of the data landscape. This super set of data feeds analytics and machine learning like never before.
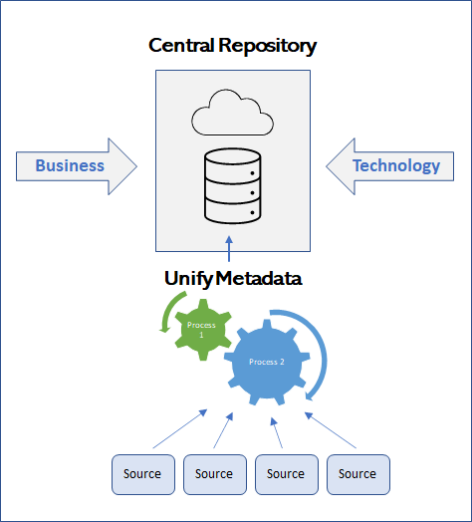
As a result, decision-making is no longer limited to descriptive analytics but more as predictive and prescriptive, meaning more data needs to be ingested. This enables the models to generate outcomes that are quicker, meaningful, and accurate. It also means data practitioners need to be more precise with their data discovery, a precursor to building reliable reports and models for trustworthy outcomes.
Impact of Metadata Management
Enter metadata management. The older “structured” taxonomy created and managed through traditional means is no longer enough. Modern management demands an intelligent means of metadata curation and the adoption into the right technology makes it possible. Three principal areas are impacted during this process.
- Auto Lineage Generation and Tracking – As the data pipelines gets created in the data lakehouse the tool should be able to capture operational data lineage down to lower granularity (column level) providing data practitioners an ability to view data flows. The technology should also keep track of audit traceability for regulatory and legal purposes.
- Auto Discover and Classify – As the data is ingested into the raw layer of the data lakehouse, the tool should be able to discover metadata and capture and document a glossary, perform semantic detection, and be able to classify, obfuscate, and protect sensitive data. In addition, you should now be able to perform associations between relevant data sets through commonly used rules.
- Enhance Cross-functional Collaboration – Data practitioners, with increased literacy using the curation the technology provides, are now able to effectively collaborate (since great data management is teamwork) while minimizing data duplicity and improving trust in data through appropriate tagging and certification. Better collaboration – better data.
A Central Catalog Means More Consistent Data
A central data catalog allows users to collaborate using metadata. This enables consistency of data accuracy, creates data congruency for quality and structure, and makes the data accessible to all users. In short, it creates an overall data literacy used across the enterprise.
“Organizations that offer users access to a curated catalog of internal and external data will realize twice the business value from analytics investments than those that do not.” “Gartner Report: Augmented Data Catalogs”
With data literacy gained through a centralized data catalog, more data practitioners (especially in non-engineering roles) also now have a mutual understanding of data assets.
Finally, when this management process is augmented through low-code/no-code technologies within an engineering and analytics realm, opportunities also open up for generalists who can be brought into the development folds to further increase developer productivity and speed. Organizations which are already struggling to on-board qualified talent will now be able to deliver more with less.

The Benefits of Metadata Management
Implementing the combination of policy and practice with all data practitioners is a challenge of leadership for certain. Doing so has many benefits for the entire management of the enterprise.
- Ease of access to information catalog through a simple interface (google search-like).
- Reduced data related risks – When metadata describes data structures clearly, such documentation reduces the risk of users selecting wrong data for reports, data sets, etc.
- Broader application support and improved reporting – in the event of a regulatory audit , a reliable reporting bolstered by a credible audit-trail is available.
- Faster response to change - one example would be to helping data professionals assemble analytic data sets quickly to study a recent guest activities, recent emergencies, or response to a data breach.
- Facilitation for broad data-sharing, in turn contributing to the success of business initiatives and processes.
- Collaboration between technical and business teams to control access and create or enforce standards
- Centralized glossaries – for cataloging, business definitions and implementation relationships, and ontologies describing relationships between objects
Impact to the Data Team
A typical data scientist spends about 85% of their time discovering and preparing (“wrangling”) data. It is challenging to understand the data and its context without proper documentation and metadata focus.

With a push towards self-service it can also mean that the catalog can assist the work of wrangling for the citizen data scientist or business user, while the more advanced IT data scientist can focus energies to ensure the models generate meaningful insights. Saves time and money.
Conclusion
Data insights can be complicated. But to truly benefit, one needs to understand their context through definitions, behavior, embedded associations through a simple searchable and centralized curated catalog. In addition, common shared knowledge into data can remove inhibitions from other corporate citizens, thereby eliminating literacy silos (not just limited to a select few SME’s) and enabling data driven conversations every day.
About the Author
Rama Ryali serves as Vice President of Product Evangelism and Strategy at RightData, a thought leader for modern data. Throughout his career, dedicated to data management and implementation, Rama served as CTO and has directed many multi-million-dollar data management initiatives. rama@getrightdata.com

About RightData
RightData is a trusted total software company that empowers end-to-end capabilities for modern data, analytics, and machine learning using modern data lakehouse and data mesh frameworks. The combination of Dextrus software for data integration and the RDt for data quality and observability provides a comprehensive DataOps approach. With a commitment to a no-code approach and a user-friendly user interface, RightData increases speed to market and provides significant cost savings to its customers. www.getrightdata.com